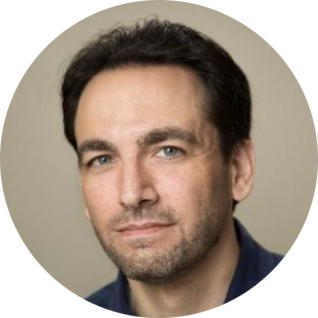
John Murphy • 7 minute read
Understanding How Important Creative Categorization is to Digital Advertising
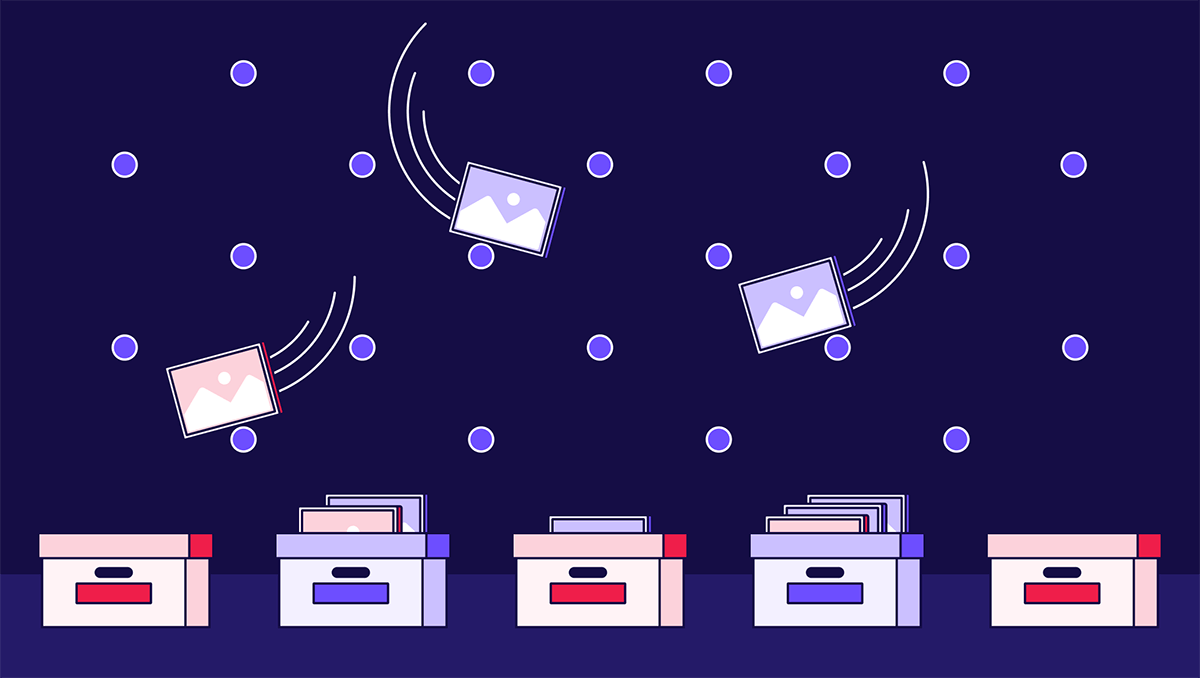
Evaluation of common approaches
At any given time, millions of unique ad creatives are active in programmatic advertising. Ensuring that each of these ads is matched with the right audience in a suitable environment is an immensely complex process that relies heavily on proper categorization. The industry’s struggles to match ads with suitable inventory, commonly referred to as “brand safety,” are well documented. But the separate problem of ensuring that suitable ads are delivered to premium publishers is less well known, although of equal importance. Publishers invest heavily in user experience, and ads that are annoying, offensive, or just a poor fit for the audience detract from that experience in ways that can damage a publisher’s reputation and lead to user churn.
For programmatic advertising channels to serve the ads that publishers want and suppress those that they don't, it is essential to accurately categorize creatives and identify potential risks. Unfortunately, the most common approaches are often inefficient and prone to errors, resulting in unreliable creative classification as well as unnecessary risks and complaints. Manual and automated classification methods each come with benefits and drawbacks, but of all the most common industry approaches to categorization, how do you know what is the right balance for your business? To find the answer, we have to consider the challenges and merits of each.
Challenges
Some of the key challenges in the categorization of creatives are fairly clear-cut, while others are a bit more opaque:
- Subjectivity and Interpretation: Subjectivity can make it challenging to establish clear and consistent categorization criteria. Ad categorization often involves a judgment call, and that judgment can vary among individuals within a team or organization based on a multitude of factors. What might be considered sensitive to one person could be completely fine to another. How do you ensure consistent categorization in such an environment? This subjectivity makes it essential for teams to establish clear and consistent categorization criteria so that individual judgements may be minimized.
- Evolving Formats and Technologies: Digital advertising is constantly evolving, with new formats and technologies emerging regularly. Categorization systems need to adapt to accommodate these changes. This requires keeping up with the latest trends and understanding how to classify ads in emerging formats like interactive ads, video ads, augmented reality (AR), virtual reality (VR), and various social media platforms.
- Blurring Boundaries: Traditional categorization systems often have predefined categories such as product/service type, target audience, or industry. However, digital ads often blur these boundaries by combining elements from different categories or using unconventional approaches. Categorizing such ads can be challenging when they don't fit neatly into existing classification schemes.
- Localization and Cultural Sensitivity: Digital ads are displayed globally, and what might be considered creative or appropriate in one culture may not be perceived the same way in another. Categorizing ads requires considering cultural nuances, language variations, and local sensitivities. Adapting categorization systems to be culturally sensitive and applicable across diverse markets globally presents new challenges.
- Suitability for Minors: As an increasing number of channels and platforms cater to minors or specifically target them as audience segments, it is crucial to evaluate the suitability of advertisements based on the presence of minors in the audience. Additionally, it is important to ensure compliance with legal obligations pertaining to the display of certain ad categories to minors, such as nudity, sexual content, alcohol, or drugs.
- Algorithmic Complexity: As the volume of digital ads increases, manual categorization by individuals becomes impractical. Automated systems leveraging machine learning algorithms are often employed. However, building accurate and robust algorithms to categorize ads requires extensive training data, addressing biases, and continuous improvement to keep up with evolving ad trends.
Overcoming these challenges requires a combination of human expertise, ongoing research, adaptability, and collaboration between creative professionals, data scientists, and marketing specialists. It involves continuously refining categorization criteria, leveraging technology, and maintaining a flexible framework to accommodate the dynamic nature of digital advertising.
A note on taxonomies
Many platforms have developed proprietary taxonomies to more accurately describe creative characteristics, but the differences between these taxonomies as well as the common practice of translating these to and from the IAB Content Taxonomy can lead to over- and under-blocking of creatives. Additionally, OpenRTB lacks a brand taxonomy, relying instead on adomain and bundle to identify an advertiser. This introduces challenges and makes completeness elusive when dealing with the millions of advertisers active in programmatic channels worldwide, including large global advertisers who might have hundreds or indeed thousands of URLs (e.g. Procter & Gamble).
The widespread use of IAB Content Taxonomy v1 as a common language is a particular source of error and confusion. While the IAB Content Taxonomy v1 has become sort of a lingua franca for the industry, it has two major drawbacks. First, the v1 taxonomy has been deprecated by IAB. Second (and more importantly), the Content Taxonomy was not designed for classification of ad creatives, but instead for classification of websites. It is missing major general categories like “car rentals” and “toys” and risk categories such as “cannabis”1. The IAB taxonomy best suited for ad classification—the IAB Ad Product Taxonomy— has not seen widespread adoption, an unfortunate outcome given its inherent superiority for the task of categorization for ads.
We realize, however, that many publishers and platforms will continue to want to use the IAB Content Taxonomy, and we provide mapping files that match our proprietary values to their closest equivalent in the various IAB taxonomies. We will also intend to support the IAB Ad Product taxonomy as it achieves greater adoption.
Evaluation of common data sources and techniques
When evaluating common data sources and techniques for ad classification, there are several important elements to consider along with their pros and cons:
Landing page URL
The landing page URL is a highly accurate and reliable source for brand and category information as well as creative risk. By examining the URL, one can gather insights into the nature of the content or product being promoted, which can help assess the level of risk associated with it.
However, isolating the actual URL from click trackers or URL shortening services can be challenging, and additional work may be necessary to extract brand and category information. And, while the landing page URL can provide valuable insights into brand and category, it may not capture all the creative-specific visual risk factors. Visual elements, such as imagery and text, play a significant role in assessing creative risk, and may not be apparent solely from the URL.
adomain (OpenRTB field)
The "adomain" field in OpenRTB is the primary method for ad categorization in use today, but it carries certain advantages and disadvantages. On the positive side, the "adomain" field is generally an accurate reflection of the brand’s website, making it a valuable and trustworthy data point.
However, the "adomain" field can be susceptible to spoofing or manipulation by advertisers or bad actors, who may misrepresent the actual brand or category. This undermines the reliability of the information derived from the field. Extracting brand and category information from the "adomain" field also requires additional work and accurate interpretation, potentially involving parsing and analyzing the domain name, which can introduce error and risk.
Moreover, relying solely on the "adomain" field may result in missing important visual risk factors associated with the ad. Elements such as text and imagery contribute significantly to creative risk, but these factors cannot be inferred solely from the domain information.
In summary, the "adomain" field in OpenRTB is often a strong signal of an ad’s brand and category, and a reasonable indication of creative risk. However, caution should be exercised due to the potential for spoofing or manipulation by bad actors. Extracting brand and category information may require additional effort, and visual risk factors cannot be captured through the "adomain" field alone.
Creative imagery
Creative imagery can provide important details beyond what can be gleaned from the landing page or an ad’s text. It enables the identification of risk factors that are orthogonal to the creative category, such as detecting the presence of alcohol in a travel advertisement. This allows for a more comprehensive assessment of potential risks associated with the ad content.
Logo detection within creative imagery can also play a crucial role in brand assignment, especially in cases where multiple brands are present. For instance, an ad for a P&G product available on Amazon might contain logos for both brands, and this co-branding might not be detectable if only the adomain or the landing page are considered. Accurate identification of co-branding is particularly important when enforcing brand blocklists, as in some cases, one brand might be fine, while the other violates the publisher’s preferences.
However, it's important to note that creative imagery has limitations as a standalone categorization element. It is unreliable as a single source of information about the product or service being advertised. While visual elements can evoke certain emotions or convey a particular theme, they may not accurately reflect the actual offerings or features of the advertised product or service.
In addition, creative imagery has low coverage, meaning that not all ads may contain distinctive or recognizable visuals for effective categorization. Moreover, there is a high false positive rate associated with image analysis techniques, leading to the potential misclassification of ads or incorrectly identifying certain elements within the imagery.
Therefore, while creative imagery can provide valuable insights into risk factors and brand assignment, it should be used in conjunction with other data sources and techniques to ensure a more comprehensive and accurate categorization of ads.
Creative text
Creative text, which is pulled from a creative using techniques like Optical Character Recognition (OCR), is another useful source of ad classification data, offering potential insights into the category, brand, or risk factors associated with an ad.
However, it's important to note that ads often have limited text, which can restrict the effectiveness of textual analysis. Many ads rely heavily on visual elements rather than textual information, making it challenging to extract meaningful insights from the text alone.
Additionally, the use of OCR for ad classification comes with an elevated false positive rate. OCR algorithms may encounter difficulties in accurately interpreting and extracting text from creatives, leading to potential misclassifications.
In summary, while creative text can offer some value in ad classification, its effectiveness is often constrained by the limited amount of text in ads and an increased risk of false positives. Complementing this element with other data sources and techniques is advisable for more comprehensive and accurate ad classification.
Advertiser self-classification
Some platforms rely entirely on advertisers to self-declare brand, category, and risk parameters. While this has the advantage of low cost, the quality of the work is often poor and major risks can be missed. Keep in mind that threat actors have little to no qualms about entering false information about themselves and their creatives. For these reasons, many SSPs ignore classification information passed from DSPs using the cat and attr parameters, choosing instead to independently confirm these values using the adomain, landing page, or other techniques.
Manual classification
Manual classification by trained raters can be a highly accurate element in ad classification. When performed by knowledgeable personnel who understand the criteria and guidelines, manual classification can provide extremely reliable results.
However, the consistency of manual classification heavily depends on the level of training and clarity of guidelines provided to the individuals. Without proper training and clear instructions, there can be inconsistencies and subjective interpretations, leading to variations in the classification outcomes.
One major drawback of manual classification is its slow, expensive, and non-scalable nature. The process requires significant human resources, time, and financial investment. As the volume of ads increases, manual classification becomes impractical and inefficient for large-scale operations. Given the expense involved, manual classification is best reserved for training machine-learning models rather than for the day-to-day work of reviewing new creatives.
In summary, while manual classification by trained individuals can offer highly accurate results, it is important to ensure consistent training and clear guidelines to minimize variations. Regardless, due to its slow, expensive, and non-scalable nature, manual classification may not be feasible or efficient to process the large number of ads flowing through programmatic channels.
While finding the ideal combination of approaches to classification can be challenging, by working with our partners to understand their sensitivities and requirements, we can adapt our approaches to best fit their needs. The process of finding the optimal balance is what Confiant excels at and continues to strive for.
1 The limitations of IAB Content Taxonomy v1 for creative classification are too numerous to detail here. The most problematic gap is the lack of nodes to describe common creative topics like Car Rental, Toys, Banking, Restaurants, Consumer Electronics, Tobacco, Gambling, Cannabis, among others. In addition, IAB has deprecated v1.This has led to a profusion of extensions and company-specific guidance (“to block gambling, set bcat equal to IAB9-7: Hobbies & Interests/Card Games”) that further confuse matters.
Not part of the Confiant customer community yet? Request a free trial today.